Remember how digital cameras changed the world of photography, images, and ads? Remember the revolution that came in terms of labor and cost-efficiency? As Artificial Intelligence and Machine Learning develop, content design processes become simpler, and more affordable.
3D animations and product rendering are increasingly demanded to launch new products and test business ideas. On the other hand, the devices we use on a daily basis are constantly improved to provide realistic experiences, be it to discover new products, compare purchase options, and understand in more detail every feature and function.
At Content2Sell we make product renders and 3D animated videos, among many other types of content. And yet, we have barely talked about this. So, we had to start somewhere.
What is Product Rendering?
Product Rendering is a process in which a designer creates an image of a product from every one of its angles, to give 360º views and depict every function through a 3D representation.
But as the worldwide growth ecommerce paves the way for product innovation, new ways to present, explain, and advertise products in more engaging ways are necessary. This is why Google launched Swirl in 2019, after seeing how 3D product frames or just 3D videos slowly establish in some websites, ecommerce and crowdfunding platforms like Kickstarter or Indiegogo.
Why use Product Rendering?
Product Rendering has a strong visual impact thanks to huge level of detail. This is why it is broadly used for educational and marketing purposes.
The high cost of product Rendering has been a problem for medium and small enterprises who could not afford the time or the money to use 3D to promote their products. Thus, it was mostly reserved for corporations and large institutions with the technological resources to benefit from them.
This is due, for the most part, for the costly effort it takes to make a virtual version with rich details, from a physical product.
Next, we’ll briefly explain how designers craft product renders.
How is Rendering done?
Before being manufactured, products need to be designed. The same happens with packaging.
Until not too long ago, designers and engineers worked on technical drawings before being able to create a prototype, manufacture it and test it. But the rapid spread of 3D design and 3D printing has accelerated, and more importantly, reduced the cost of such a long process.
Rendering from 3D Source files
One of the simplest ways to render a product is from the source file, usually provided by the product’s designer. However, those source files need to be processed by graphic designers, who will choose the right materials, textures, and colors to transform it into a hyper realistic 3D image or animation.
Reproduction by designers
As said above, working on technical drawings is expensive and time-consuming. And yet, some industries continue to use them.
When no 3D file is available, someone must create it. Sometimes, from a technical drawing, and some other times, from the physical product itself. The latter means drawing every line and surface of the product in the 3D software. And that is, needless to say, a very hard work.
Multi-angle pictures / Scanners
An easier way to make product renders is taking pictures from different angles: top, bottom, left and right. This also involves disassembling the product and all of its parts, to create a 3D model and then adding animations. This allows making exploded views, 360º interactive presentations, and mixes with real-life videos to provide context and highlight specific features.
The NeRF Revolution
Nvidia has recently announced a new feature in its graphic cards that allows 2D pictures to become 3D, making it more affordable for smaller companies. To do this, a new technology in some of its graphic cards use Artificial Intelligence to speed up the process.
We won’t get too technical on this.
NeRF stands for Neural Radiance Fields. An evolution of Ray Tracing (tracing the light reflection in each pixel until its source) and Volume Ray Tracing (which added volumetric information in every pixel, turning it in a Voxel). As the acronym indicates, the main innovation is a neural network within the Graphic Processing Units that is able to calculate how light reflections change in every position, according to the materials, their textures, shapes, and shades.
As of today, this is not possible with standard cameras. Instead, several cameras are necessary for optimal results, as well as some specific circumstances. In other words, we shouldn’t expect to capture a fast-moving subject in 2D and obtain a 3D image.
The video released by the company shows how four photos taken from different points of view merge into a three-dimensional image.
Nvidia’s innovation refers to the speed at which Artificial Intelligence makes the calculations to achieve this. In this vein, we should recall that a computer is absolutely necessary to process all that information, and that the graphics cards capable of this are only in professional cameras using Tensor Core technology.
Tensor cores are graphic processing units (GPU) designed to multiply matrixes 12 times faster than its predecessors.
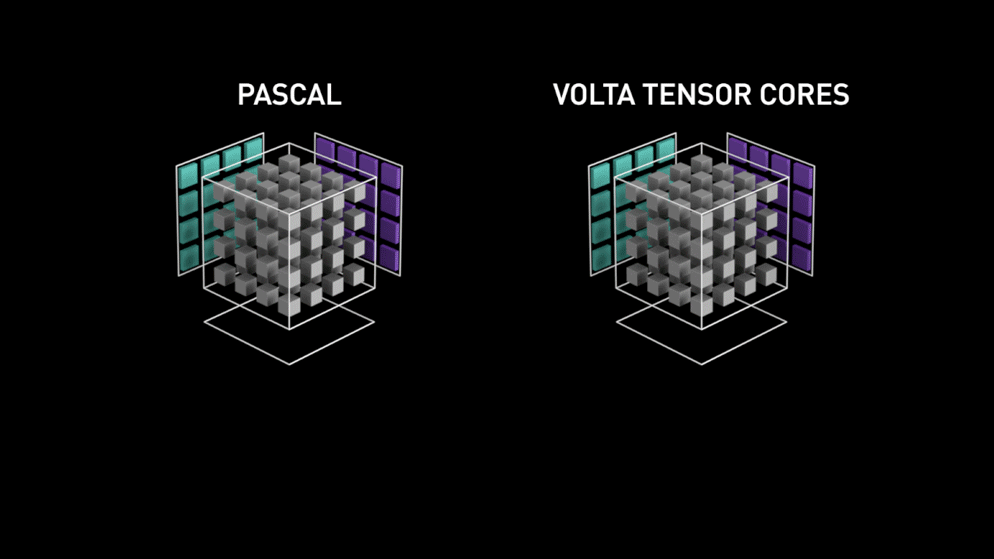
According to the company, NeRF will help training robots and autonomous cars, to understand the size and shape of real-world objects through 2D image captures or video sequences. Also in architecture and entertainment to generate digital representations of real environments that creators can modify. Easier, faster, and with a much lower consumption.
Not a final, but a necessary step
The NeRF (Neural Radiance Fields) technology is, in any case, a big step forward. By using deep learning techniques, it opens the door for anyone with a relatively affordable computer to make 3D images on the spot. NeRF’s main technical advance is the calculation speed to keep both the sharpness of the photographed object / person, and the depth of the area. But of course, because Artificial Intelligence needs to learn, we must give it time before seeing how effective it is in practice. This is only the beginning. seems to be still in the development phase. There is a lot of mystery around how effective Deep Learning can be, and, and how long it can take to deliver the expected results.